Imagine you’re working at a tech company trying to boost user engagement on its website.
You decide to test a small change: making the signup button slightly bigger. With a traditional A/B testing approach, you might show half of your incoming traffic—group A—the new, larger button, while keeping the button the same for the rest—group B—for about a week. Then, you’d compare the conversion rates of the two groups to determine if the bigger button made a difference.
This approach produces useful data on whether this change improves user engagement. But what if you now want to try a different font or a different color? The right subtle change could significantly affect traffic and conversions over time. However, experimenting with various options would require many A/B tests, requiring valuable time and resources. In addition, you risk losing traction from a large set of users during the testing period if you test a bad variant.
A/B testing shines in settings with an asymmetrical utility function—high-stakes settings where the cost of picking the wrong option is significant—like choosing a vaccine. If a health organization were to select the wrong vaccine, there might be a high cost to switch to the correct one. But in the world of website UI and copy, the stakes are different. Adjusting the signup button size is a far easier fix than recalling a vaccine. Here, the focus is on creating the most optimal design rather than precise measures of uncertainty.
Enter Multi-Armed Bandits (MABs), the statistical technique at the heart of Coframe’s approach.
How exactly do MABs differ from A/B testing? Think of it like this: you’re in a casino with thousands of slot machines, each with slightly different odds of winning. Instead of limiting yourself to two machines and switching back and forth between them to find the better one, you employ a smart assistant. This assistant suggests machines for you to try and keeps track of which ones perform well. Ultimately, it balances exploitation and exploration—maximizing your winnings from the machines you know work while still seeking out new, potentially better options from the casino’s massive selection.
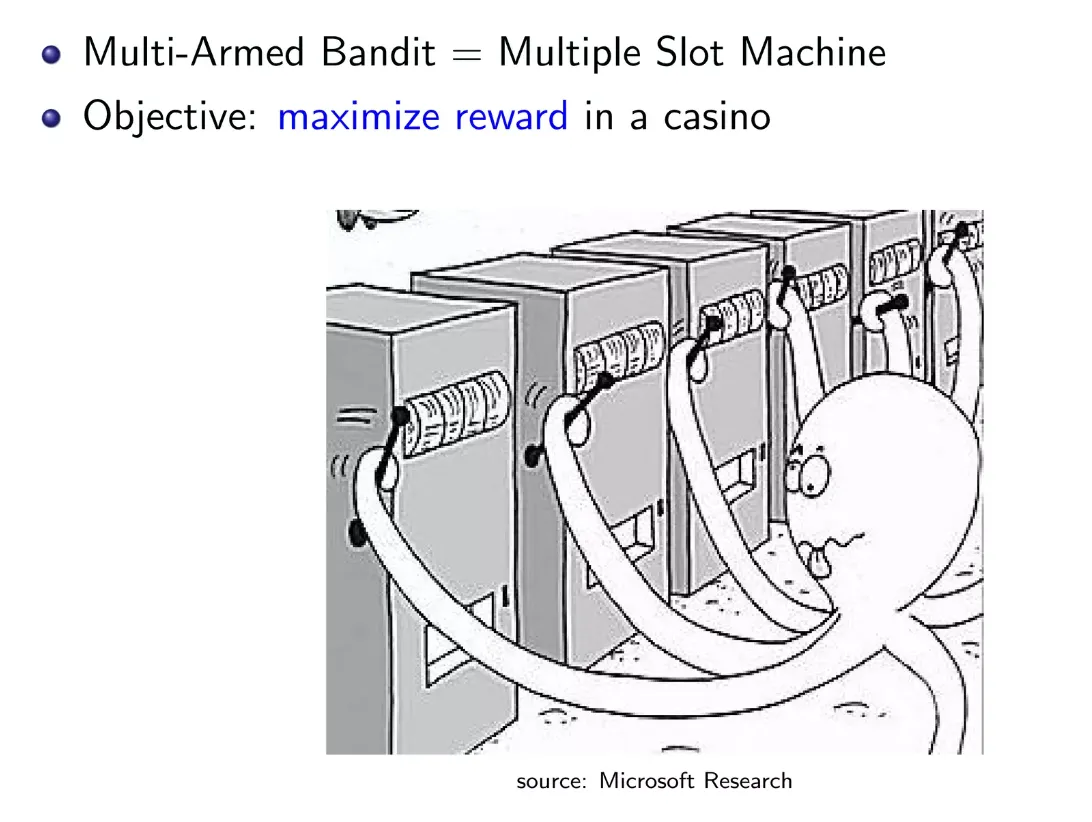
While A/B testing is a tried-and-true technique, it’s inherently limited: it’s restricted to two static options and only delivers meaningful results after a lengthy testing period.
On the other hand, MABs, like your casino assistant, are optimization processes. Rather than being restricted to testing just two candidates at a time, MABs continuously test several “arms”—like different versions of a signup button or several phrasings of copy text—while tracking their performance. As users visit the site, MABs dynamically allocate traffic to different variants: more users are directed towards versions that have historically performed better, while some are occasionally shown new or less-tested variants.
This offers several advantages over traditional A/B testing. First, MABs allow you to quickly explore multiple variants in parallel, without having to wait for the results of dozens of A/B tests. Second, your website is optimized during the testing process: unlike in traditional A/B testing, traffic is directed to the better variant almost immediately, meaning there is a lower risk of losing engagement by continuing to serve a poor-performing variant.
So, in summary, while A/B testing is a good measure of uncertainty and provides clear statistical results, it’s limited to comparing two options over a long period of time. On the other hand, MABs are more flexible and efficient: they can test a wide array of options simultaneously, optimizing user experience quickly while also collecting data on the most effective variants.